Deep Dive into RAG 2.0: A Technological Leap in AI
In the realm of artificial intelligence, March 2024 marked a significant milestone with the introduction of RAG 2.0, a sophisticated AI system designed for enterprise-grade applications. Beyond its initial announcement, RAG 2.0 stands as a testament to the power of end-to-end optimization and the integration of advanced Contextual Language Models (CLMs). This article delves into the technical intricacies and innovations that set RAG 2.0 apart, shedding light on its potential to redefine the standards of generative AI.
End-to-End Optimization: The Core of RAG 2.0
At its heart, RAG 2.0 revolutionizes AI systems through end-to-end optimization. Unlike traditional methods that optimize components in isolation, RAG 2.0 treats the language model and retriever as a cohesive unit. This holistic approach allows for direct feedback between the language generation and information retrieval processes, significantly enhancing the system's ability to produce accurate and contextually relevant responses. The benefits are multifold, including increased efficiency and a marked reduction in the discrepancies that can arise from independently optimized components.
Bridging Components: The Synergy of Language Models and Retrievers
A critical innovation within RAG 2.0 is the seamless integration of the language model and retriever. This integration is achieved through sophisticated algorithms that enable real-time interaction between the retrieval of relevant information and its subsequent synthesis into coherent responses. By employing techniques such as gradient backpropagation, RAG 2.0 aligns the objectives of both components, ensuring that the retriever's output directly influences the language model's training. This synergy not only improves the accuracy of generated content but also significantly enhances the model's ability to adapt to new or evolving information domains.

Contextual Language Models: The Backbone of RAG 2.0
Contextual Language Models (CLMs) represent a cornerstone of RAG 2.0's technological prowess. Unlike standard language models, CLMs are designed to understand and leverage context more effectively, allowing for more nuanced and accurate generation of text. The training process of CLMs involves a sophisticated regime that integrates vast datasets with contextual cues, enabling the model to discern and replicate intricate patterns of human language. This process ensures that CLMs can generate responses that are not only relevant but also deeply rooted in the context provided, setting a new benchmark in natural language understanding and generation.
Benchmarking Excellence: RAG 2.0's Performance Metrics
RAG 2.0's superiority is evidenced by its performance across a variety of benchmarks. By employing exact match metrics in domains such as open-domain question answering, RAG 2.0 demonstrates its unparalleled ability to retrieve and utilize information accurately. Moreover, in benchmarks focusing on the model's ability to generate faithful and contextually grounded responses, RAG 2.0 outshines existing models. These benchmarks serve as a critical measure of an AI system's efficacy in real-world applications, affirming RAG 2.0's leading position in the field.
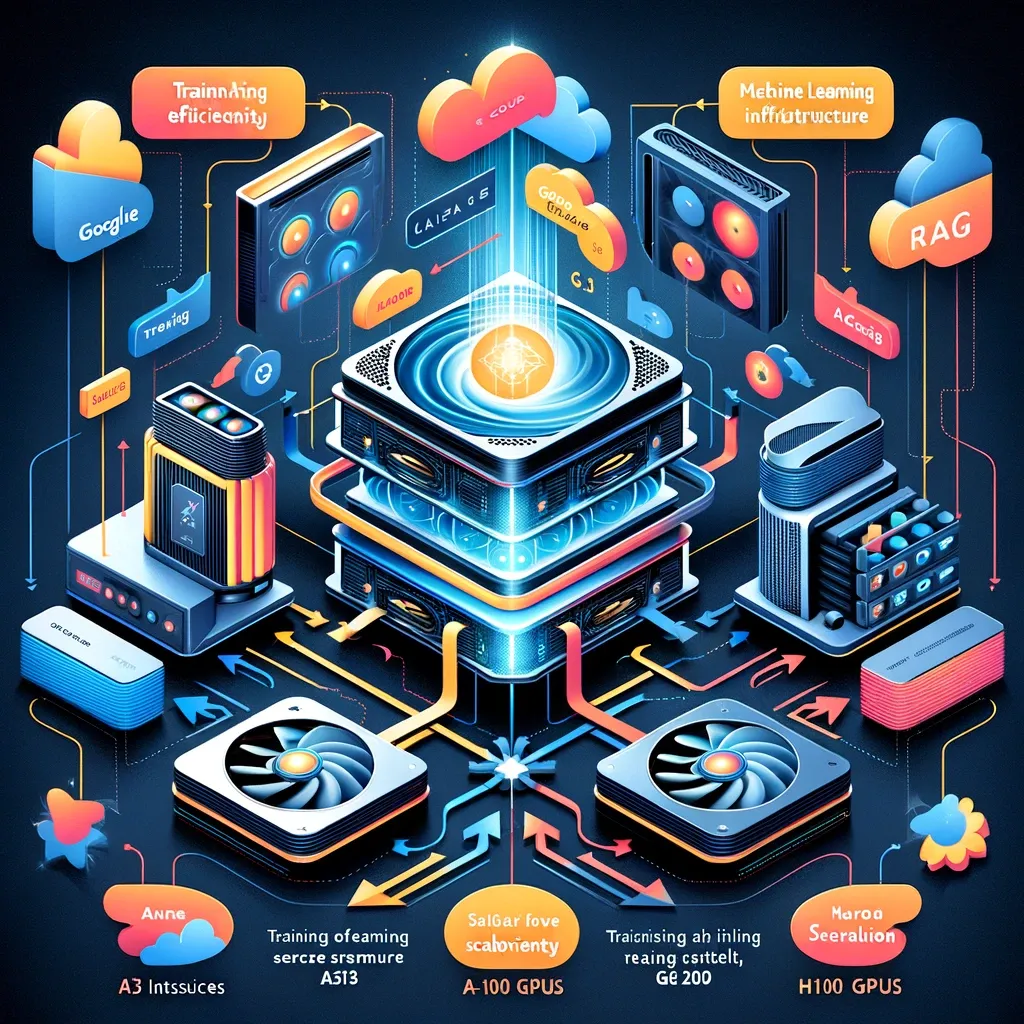
Beyond Theory: RAG 2.0 in Real-World Applications
The practical applications of RAG 2.0 extend across various sectors, from finance and law to engineering, showcasing its adaptability and effectiveness. Through detailed case studies, such as its application in financial analysis, RAG 2.0 has proven capable of navigating complex, domain-specific queries with remarkable accuracy and efficiency. These real-world implementations highlight RAG 2.0's potential to transform industry practices by providing AI solutions that are not only powerful but also highly reliable.
The Technical Backbone: Infrastructure and Scalability
The deployment and training of RAG 2.0 are supported by the latest advancements in machine learning infrastructure, including Google Cloud's A3 instances equipped with H100 GPUs. This cutting-edge infrastructure underpins RAG 2.0's training process, enabling the handling of vast datasets with unprecedented efficiency. The choice of hardware and networking solutions plays a pivotal role in RAG 2.0's scalability and performance, ensuring that the system can meet the demands of enterprise-level applications without compromising on speed or accuracy.
In summary, RAG 2.0 represents a paradigm shift in the development of generative AI, driven by technical innovations in end-to-end optimization, the integration of language models and retrievers, and the pioneering use of Contextual Language Models. Its proven performance across benchmarks and real-world applications underscores the potential of RAG 2.0 to set new standards in AI, promising a future where AI systems are not only more efficient and reliable but also capable of understanding and generating human language with unprecedented accuracy.